how-editors-can-increase-reproducibility-and-transparency
December 11, 2019
The process of doing research can be a messy business and reproducing research can be challenging too. In a survey of over 1,500 researchers in 2016, Nature found that 52% felt that there were significant concerns about reproducibility. This finding is also echoed by the failure to replicate results in preclinical cancer research, social sciences and psychology among other fields.
Reproducibility (obtaining the same results using the same data) and replication (obtaining consistent results from new data) are key issues for research and concern everyone – funders, researchers, institutions, and journals alike. We highlighted a range of solutions to increase reproducibility and transparency, including embracing the Transparency and Openness Promotion “TOP” guidelines; use of transparent reporting guidelines and checklists; the Registered Reports initiative; and better training and practices. However, given recent funding initiatives, especially with regard to open access to data in Horizon Europe, we were particularly keen to hear editors’ views with respect to another solution: data sharing. And so that’s what we spent the rest of the workshop exploring.
"Reproducibility of research can be improved by increasing transparency of the research process and products." - Brian Nosek, Executive Director, Center for Open Science |
To gauge the temperature in the room with respect to data sharing, we asked editors to move to the right of the room if they were hot about data sharing for their journal, to move to the left if they were cold, and to move to the middle if they were lukewarm. It was really encouraging to see that most editors moved to the right of the room.
In exploring some of the potential concerns that made people slightly cold or lukewarm about data-sharing, editors voiced several issues: “How to handle sensitive or commercial data?” “What about the resources and time needed for proper curation?” “What do we mean by data – raw data or final data?” “How to handle big datasets?” “What is meant by open data?”
We recognise that opening up data, or to be explicit, sharing FAIR data (data that is Findable, Accessible, Interoperable, and Reusable – as discussed in this primer) is a significant challenge and a “one size fits all” solution to data sharing does not work for all research communities or disciplines.
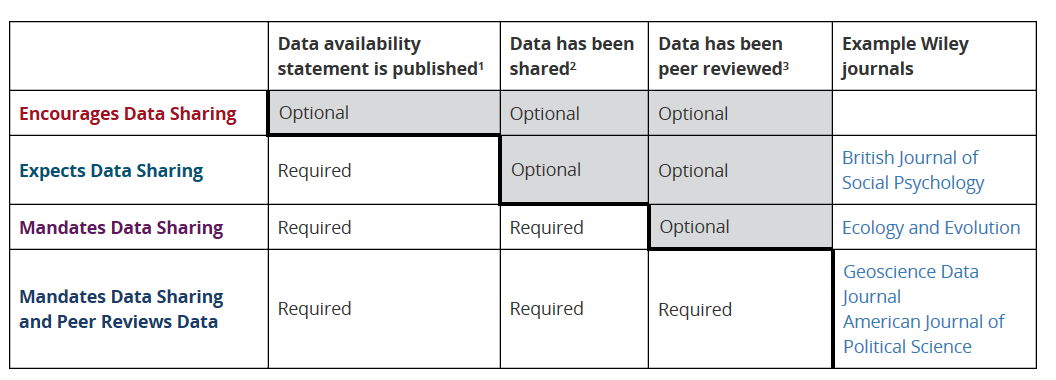
We explained Wiley’s approach to data sharing through developing policies, in line with other publishers and the Research Data Alliance, that offer options for research communities with four key policies summarized below and explained in more detail here.
Wiley’s data sharing policies as outlined here.
We reasoned that it is a first manageable step for a journal that currently is encouraging data sharing to move to our “expects data” policy (i.e. by including in their research article a data availability statement that says something about the data, what it is, and where it can be found even if they do not necessarily share the data).
So, if you are an editor reading this post, we’d like you to reflect on whether you would be willing to take a step forward on your data sharing journey by implementing our “expects data” policy for your journal. The only requirement for authors is to disclose whether or not they’ve shared new data in a data availability statement. We have a toolkit that makes implementing the policy straightforward, please speak to your Journal Publishing Manager if you are interested. Finally, a huge thank you to the editors who took part in the discussion at the workshop. We hope that we can encourage transparency and reproducibility of research outputs together by embracing a data policy that, at the very least, means that researchers will explain what their data is and where it can be found.